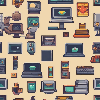
Why is ResNet the best?
Could you elaborate on why ResNet is often considered the best among its peers in the field of neural networks? What specific characteristics or advantages does it possess that sets it apart from other architectures? Are there any benchmarks or real-world applications where ResNet has demonstrated exceptional performance, and if so, could you provide some examples? As a practitioner in the field, what are your thoughts on why ResNet has gained such widespread popularity and acceptance?
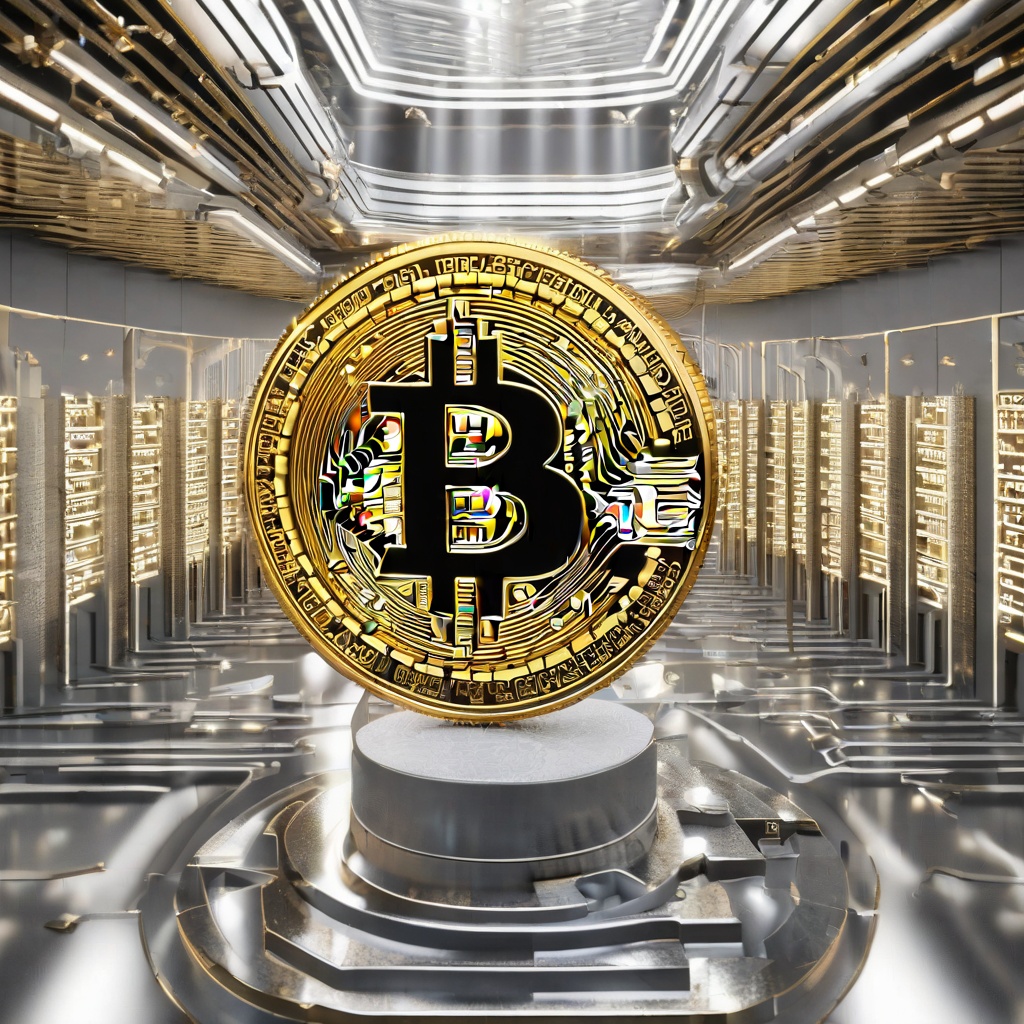
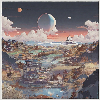
What is the weakness of ResNet?
Could you elaborate on the potential drawbacks or limitations of the ResNet architecture in the realm of deep learning and neural networks? Specifically, are there any scenarios where ResNet might struggle to achieve optimal performance or face challenges that other network architectures might handle more effectively? It would be insightful to understand the weaknesses that researchers and practitioners should be aware of when utilizing ResNet for various applications.
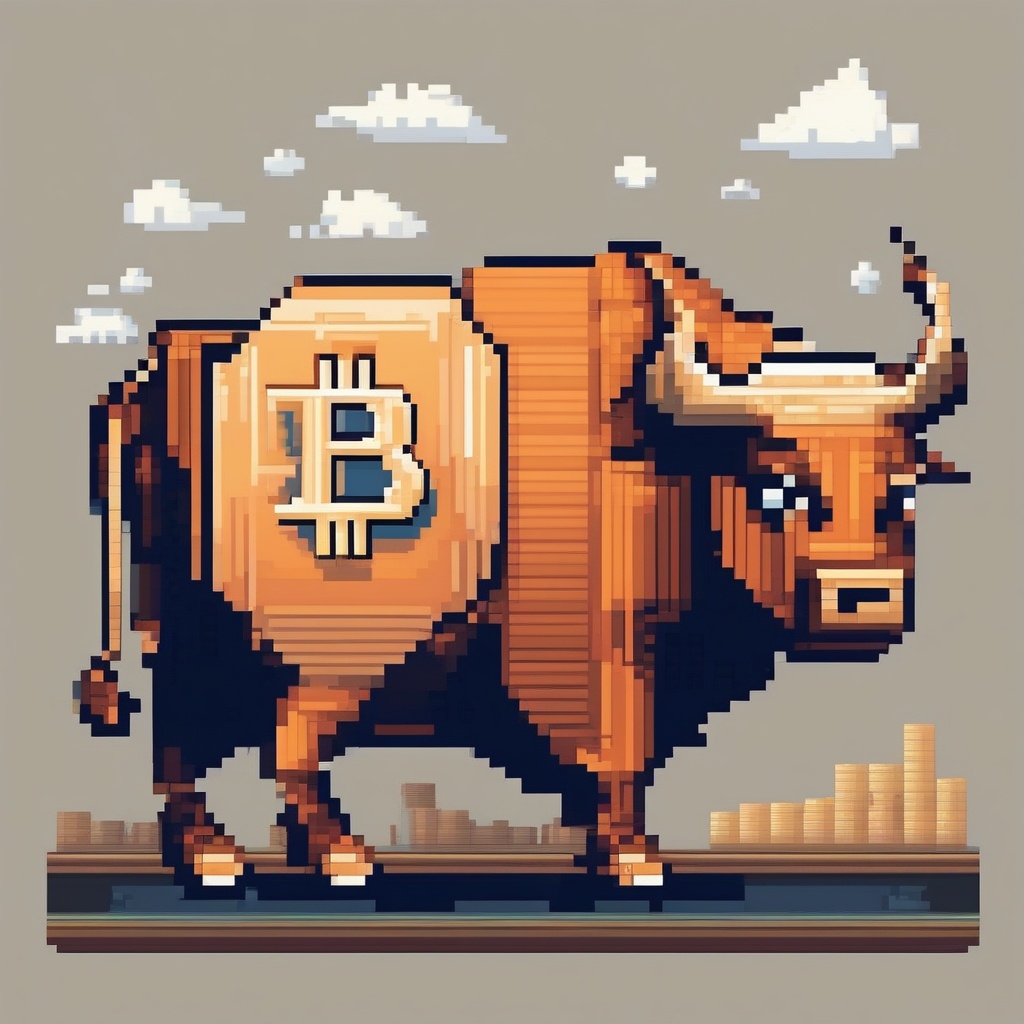
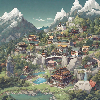
Is ViT better than ResNet?
Could you elaborate on why you're asking if Vision Transformer (ViT) is better than Residual Network (ResNet)? Both models have their unique strengths and are suitable for different tasks in computer vision. ViT, for instance, excels at capturing global context and long-range dependencies, while ResNet is known for its ability to handle complex patterns and deep hierarchical representations. Are you looking for a model that can achieve better performance on a specific task, or are you interested in understanding the fundamental differences between the two architectures?
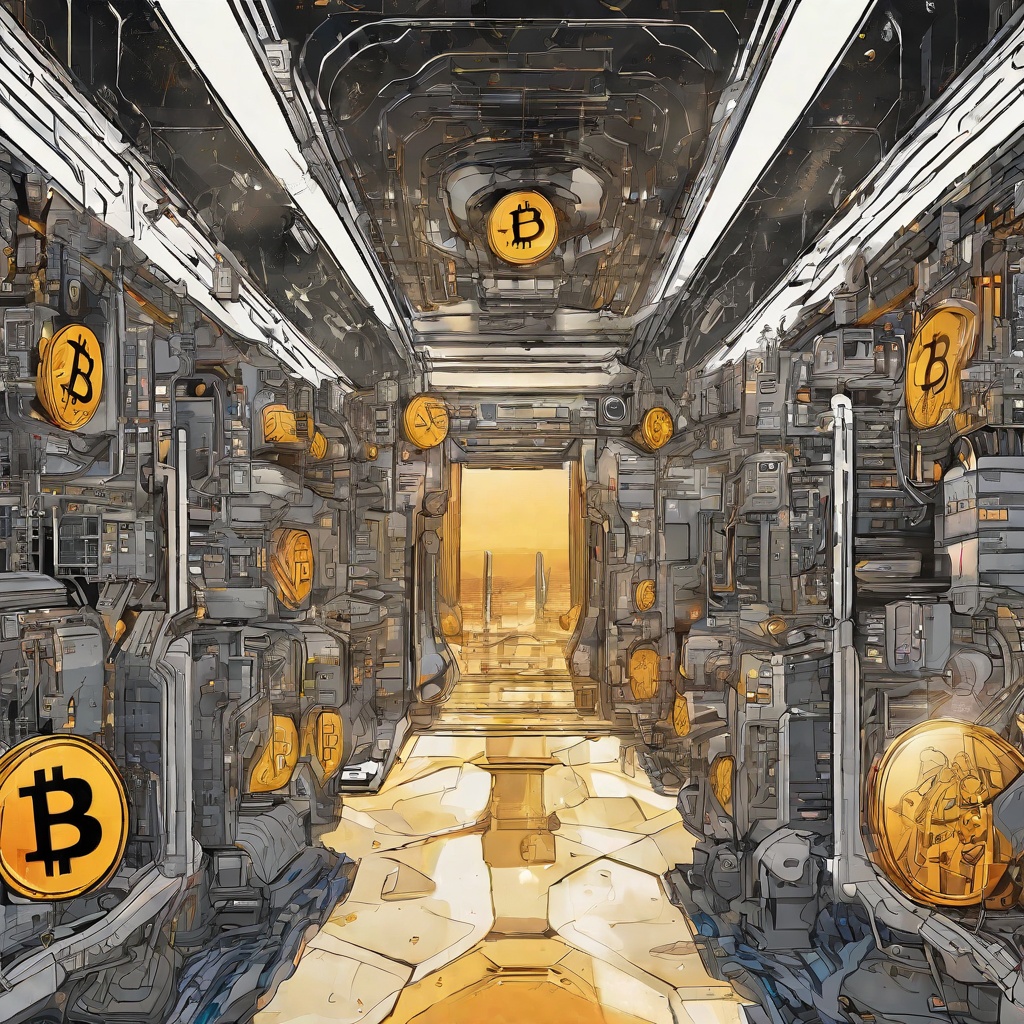